diff --git a/_posts/2017-07-11-generating-random-poems-with-python.md b/_posts/2017-07-11-generating-random-poems-with-python.md new file mode 100644 index 0000000..5626e1a --- /dev/null +++ b/_posts/2017-07-11-generating-random-poems-with-python.md @@ -0,0 +1,514 @@ +--- +title: Generating Random Poems with Python +layout: post +hidden: true +--- + +In this post, I will demonstrate how to begin generating random text using a few +lines of standard python and then progressively refining the output until it +looks poem-like. + +If you would like to follow along with this post and actually run the code +snippets mentioned here, you can clone [my NLP +repository](https://github.com/thallada/nlp/) and run [the Jupyter +notebook](https://github.com/thallada/nlp/blob/master/edX%20Lightning%20Talk.ipynb). + +You might not realize it, but you probably use an app everyday that can generate +random text that sounds like you: your phone keyboard. + + + +So how does it work? + +## Corpus + +First, we need a **corpus**: the text our generator will recombine into new +sentences. In the case of your phone keyboard, this is all the text you've ever +typed into your keyboard. For our example, let's just start with one sentence: + +```python +corpus = 'The quick brown fox jumps over the lazy dog' +``` + +## Tokenization + +Now we need to split this corpus into individual **tokens** that we can operate +on. Since our objective is to eventually predict the next word from the previous +word, we will want our tokens to be individual words. This process is called +**tokenization**. The simplest way to tokenize a sentence into words is to split +on spaces: + +```python +words = corpus.split(' ') +words +``` +```python +['The', 'quick', 'brown', 'fox', 'jumps', 'over', 'the', 'lazy', 'dog'] +``` + +## Bigrams + +Now, we will want to create **bigrams**. A bigram is a pair of two words that +are in the order they appear in the corpus. To create bigrams, we will iterate +through the list of the words with two indices, one of which is offset by one: + +```python +bigrams = [b for b in zip(words[:-1], words[1:])] +bigrams +``` +```python +[('The', 'quick'), + ('quick', 'brown'), + ('brown', 'fox'), + ('fox', 'jumps'), + ('jumps', 'over'), + ('over', 'the'), + ('the', 'lazy'), + ('lazy', 'dog')] +``` + +How do we use the bigrams to predict the next word given the first word? + +Return every second element where the first element matches the **condition**: + +```python +condition = 'the' +next_words = [bigram[1] for bigram in bigrams + if bigram[0].lower() == condition] +next_words +``` +```python +['quick', 'lazy'] +``` + +We have now found all of the possible words that can follow the condition "the" +according to our corpus: "quick" and "lazy". + +
+(The quick) (quick brown) ... (the lazy) (lazy dog) ++ +Either "quick" or "lazy" could be the next word. + +## Trigrams and N-grams + +We can partition our corpus into groups of threes too: + +
+(The quick brown) (quick brown fox) ... (the lazy dog) ++ +Or, the condition can be two words (`condition = 'the lazy'`): + +
+(The quick brown) (quick brown fox) ... (the lazy dog) ++ +These are called **trigrams**. + +We can partition any **N** number of words together as **n-grams**. + +## Conditional Frequency Distributions + +Earlier, we were able to compute the list of possible words to follow a +condition: + +```python +next_words +``` +```python +['quick', 'lazy'] +``` + +But, in order to predict the next word, what we really want to compute is what +is the most likely next word out of all of the possible next words. In other +words, find the word that occurred the most often after the condition in the +corpus. + +We can use a **Conditional Frequency Distribution (CFD)** to figure that out! A +**CFD** can tell us: given a **condition**, what is **likelihood** of each +possible outcome. + +This is an example of a CFD with two conditions, displayed in table form. It is +counting words appearing in a text collection (source: nltk.org). + +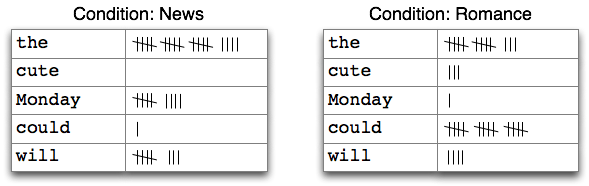 + +Let's change up our corpus a little to better demonstrate the CFD: + +```python +words = ('The quick brown fox jumped over the ' + 'lazy dog and the quick cat').split(' ') +print words +``` +```python +['The', 'quick', 'brown', 'fox', 'jumped', 'over', 'the', 'lazy', 'dog', 'and', 'the', 'quick', 'cat'] +``` + +Now, let's build the CFD. I use +[`defaultdicts`](https://docs.python.org/2/library/collections.html#defaultdict-objects) +to avoid having to initialize every new dict. + +```python +from collections import defaultdict +cfd = defaultdict(lambda: defaultdict(lambda: 0)) +for i in range(len(words) - 2): # loop to the next-to-last word + cfd[words[i].lower()][words[i+1].lower()] += 1 + +# pretty print the defaultdict +{k: dict(v) for k, v in dict(cfd).items()} +``` +```python +{'and': {'the': 1}, + 'brown': {'fox': 1}, + 'dog': {'and': 1}, + 'fox': {'jumped': 1}, + 'jumped': {'over': 1}, + 'lazy': {'dog': 1}, + 'over': {'the': 1}, + 'quick': {'brown': 1}, + 'the': {'lazy': 1, 'quick': 2}} +``` + +So, what's the most likely word to follow `'the'`? + +```python +max(cfd['the']) +``` +```python +'quick' +``` + +Whole sentences can be the conditions and values too. Which is basically the way +[cleverbot](http://www.cleverbot.com/) works. + + + +## Random Text + +Lets put this all together, and with a little help from +[nltk](http://www.nltk.org/) generate some random text. + +```python +import nltk +import random + +TEXT = nltk.corpus.gutenberg.words('austen-emma.txt') + +# NLTK shortcuts :) +bigrams = nltk.bigrams(TEXT) +cfd = nltk.ConditionalFreqDist(bigrams) + +# pick a random word from the corpus to start with +word = random.choice(TEXT) +# generate 15 more words +for i in range(15): + print word, + if word in cfd: + word = random.choice(cfd[word].keys()) + else: + break +``` + +Which outputs something like: + +``` +her reserve and concealment towards some feelings in moving slowly together . +You will shew +``` + +Great! This is basically what the phone keyboard suggestions are doing. Now how +do we take this to the next level and generate text that looks like a poem? + +## Random Poems + +Generating random poems is accomplished by limiting the choice of the next word +by some constraint: + +* words that rhyme with the previous line +* words that match a certain syllable count +* words that alliterate with words on the same line +* etc. + +## Rhyming + +### Written English != Spoken English + +English has a highly **nonphonemic orthography**, meaning that the letters often +have no correspondence to the pronunciation. E.g.: + +> "meet" vs. "meat" + +The vowels are spelled differently, yet they rhyme. + +Fun fact: They used to be pronounced differently in Middle English during the +invention of the printing press and standardized spelling. The [Great Vowel +Shift](https://en.wikipedia.org/wiki/Great_Vowel_Shift) happened after, and is +why they are now pronounced the same. + +So if the spelling of the words is useless in telling us if two words rhyme, +what can we use instead? + +### International Phonetic Alphabet (IPA) + +The IPA is an alphabet that can represent all varieties of human pronunciation. + +* meet: /mit/ +* meat: /mit/ + +Note: this is only the IPA transcription for only one **accent** of English. +Some English speakers may pronounce these words differently which could be +represented by a different IPA transcription. + +## Syllables + +How can we determine the number of syllables in a word? Let's consider the two +words "poet" and "does": + +* "poet" = 2 syllables +* "does" = 1 syllable + +The vowels in these two words are written the same, but are pronounced +differently with a different number of syllables. + +Can the IPA tell us the number of syllables in a word too? + +* poet: /ˈpoʊət/ +* does: /ˈdʌz/ + +Not really... We cannot easily identify the number of syllables from those +transcriptions. Sometimes the transcriber denotes syllable breaks with a `.` or +a `'`, but sometimes they don't. + +### Arpabet + +The Arpabet is a phonetic alphabet developed by ARPA in the 70s that: + +* Encodes phonemes specific to American English. +* Meant to be a machine readable code. It is ASCII only. +* Denotes how stressed every vowel is from 0-2. + +This is perfect! Because of that third bullet, a word's syllable count equals +the number of digits in the Arpabet encoding. + +### CMU Pronouncing Dictionary (CMUdict) + +A large open source dictionary of English words to North American pronunciations +in Arpanet encoding. Conveniently, it is also in NLTK... + +### Counting Syllables + +```python +import string +from nltk.corpus import cmudict +cmu = cmudict.dict() + +def count_syllables(word): + lower_word = word.lower() + if lower_word in cmu: + return max([len([y for y in x if y[-1] in string.digits]) + for x in cmu[lower_word]]) + +print("poet: {}\ndoes: {}".format(count_syllables("poet"), + count_syllables("does"))) +``` + +Results in: + +``` +poet: 2 +does: 1 +``` + +## Buzzfeed Haiku Generator + +To see this in action, try out a haiku generator I created that uses Buzzfeed +article titles as a corpus. It does not incorporate rhyming, it just counts the +syllables to make sure it's 5-7-5 [as it +should](https://en.wikipedia.org/wiki/Haiku). You can view the full code +[here](https://github.com/thallada/nlp/blob/master/generate_poem.py). + + + +Run it live at: +[http://mule.hallada.net/nlp/buzzfeed-haiku-generator/](http://mule.hallada.net/nlp/buzzfeed-haiku-generator/) + +## Syntax-aware Generation + +Remember these? + + + +Mad Libs worked so well because they forced the random words (chosen by the +players) to fit into the syntactical structure and parts-of-speech of an +existing sentence. + +You end up with **syntactically** correct sentences that are **semantically** +random. We can do the same thing! + +### NLTK Syntax Trees! + +NLTK can parse any sentence into a [syntax +tree](http://www.nltk.org/book/ch08.html). We can utilize this syntax tree +during poetry generation. + +```python +from stat_parser import Parser +parsed = Parser().parse('The quick brown fox jumps over the lazy dog.') +print parsed +``` + +Syntax tree output as an +[s-expression](https://en.wikipedia.org/wiki/S-expression): + +``` +(S + (NP (DT the) (NN quick)) + (VP + (VB brown) + (NP + (NP (JJ fox) (NN jumps)) + (PP (IN over) (NP (DT the) (JJ lazy) (NN dog))))) + (. .)) +``` + +```python +parsed.pretty_print() +``` + +And the same tree visually pretty printed in ASCII: + +``` + S + ________________________|__________________________ + | VP | + | ____|_____________ | + | | NP | + | | _________|________ | + | | | PP | + | | | ________|___ | + NP | NP | NP | + ___|____ | ___|____ | _______|____ | + DT NN VB JJ NN IN DT JJ NN . + | | | | | | | | | | +the quick brown fox jumps over the lazy dog . +``` + +NLTK also performs [part-of-speech tagging](http://www.nltk.org/book/ch05.html) +on the input sentence and outputs the tag at each node in the tree. Here's what +each of those mean: + +|**S** | Sentence | +|**VP** | Verb Phrase | +|**NP** | Noun Phrase | +|**DT** | Determiner | +|**NN** | Noun (common, singular) | +|**VB** | Verb (base form) | +|**JJ** | Adjective (or numeral, ordinal) | +|**.** | Punctuation | + +Now, let's use this information to swap matching syntax sub-trees between two +corpora ([source for the generate +function](https://github.com/thallada/nlp/blob/master/syntax_aware_generate.py)). + +```python +from syntax_aware_generate import generate + +# inserts matching syntax subtrees from trump.txt into +# trees from austen-emma.txt +generate('trump.txt', word_limit=10) +``` +``` + +(SBARQ + (SQ + (NP (PRP I)) + (VP (VBP do) (RB not) (VB advise) (NP (DT the) (NN custard)))) + (. .)) +I do not advise the custard . +============================== +I do n't want the drone ! +(SBARQ + (SQ + (NP (PRP I)) + (VP (VBP do) (RB n't) (VB want) (NP (DT the) (NN drone)))) + (. !)) +``` + +Above the line is a sentence selected from a corpus of Jane Austen's *Emma*. +Below it is a sentence generated by walking down the syntax tree and finding +sub-trees from a corpus of Trump's tweets that match the same syntactical +structure and then swapping the words in. + +The result can sometimes be amusing, but more often than not, this approach +doesn't fare much better than the n-gram based generation. + +### spaCy + +I'm only beginning to experiment with the [spaCy](https://spacy.io/) Python +library, but I like it a lot. For one, it is much, much faster than NLTK: + + + +[https://spacy.io/docs/api/#speed-comparison](https://spacy.io/docs/api/#speed-comparison) + +The [API](https://spacy.io/docs/api/) takes a little getting used to coming from +NLTK. It doesn't seem to have any sort of out-of-the-box solution to printing +out syntax trees like above, but it does do [part-of-speech +tagging](https://spacy.io/docs/api/tagger) and [dependency relation +mapping](https://spacy.io/docs/api/dependencyparser) which should accomplish +about the same. You can see both of these visually with +[displaCy](https://demos.explosion.ai/displacy/). + +## Neural Network Based Generation + +If you haven't heard all the buzz about [neural +networks](https://en.wikipedia.org/wiki/Artificial_neural_network), they are a +particular technique for [machine +learning](https://en.wikipedia.org/wiki/Machine_learning) that's inspired by our +understanding of the human brain. They are structured into layers of nodes which +have connections to other nodes in other layers of the network. These +connections have weights which each node multiplies by the corresponding input +and enters into a particular [activation +function](https://en.wikipedia.org/wiki/Activation_function) to output a single +number. The optimal weights of every connection for solving a particular problem +with the network are learned by training the network using +[backpropagation](https://en.wikipedia.org/wiki/Backpropagation) to perform +[gradient descent](https://en.wikipedia.org/wiki/Gradient_descent) on a +particular [cost function](https://en.wikipedia.org/wiki/Loss_function) that +tries to balance getting the correct answer while also +[generalizing](https://en.wikipedia.org/wiki/Regularization_(mathematics)) the network +enough to perform well on data the network hasn't seen before. + +[Long short-term memory +(LSTM)](https://en.wikipedia.org/wiki/Long_short-term_memory) is a type of +[recurrent neural network +(RNN)](https://en.wikipedia.org/wiki/Recurrent_neural_network) (a network with +cycles) that can remember previous values for a short or long period of time. +This property makes them remarkably effective at a multitude of tasks, one of +which is predicting text that will follow a given sequence. We can use this to +continually generate text by inputting a seed, appending the generated output to +the end of the seed, removing the first element from the beginning of the seed, +and then inputting the seed again, following the same process until we've +generated enough text from the network ([paper on using RNNs to generate +text](http://www.cs.utoronto.ca/~ilya/pubs/2011/LANG-RNN.pdf)). + +Luckily, a lot of smart people have done most of the legwork so you can just +download their neural network architecture and train it yourself. There's +[char-rnn](https://github.com/karpathy/char-rnn) which has some [really exciting +results for generating texts (e.g. fake +Shakespeare)](http://karpathy.github.io/2015/05/21/rnn-effectiveness/). There's +also [word-rnn](https://github.com/larspars/word-rnn) which is a modified +version of char-rnn that operates on words as a unit instead of characters. +Follow [my last blog post on how to install TensorFlow on Ubuntu +16.04](/2017/06/20/how-to-install-tensorflow-on-ubuntu-16-04.html) and +you'll be almost ready to run a TensorFlow port of word-rnn: +[word-rnn-tensorflow](https://github.com/hunkim/word-rnn-tensorflow). + +I plan on playing around with NNs a lot more to see what kind of poetry-looking +text I can generate from them. diff --git a/img/blog/buzzfeed.jpg b/img/blog/buzzfeed.jpg new file mode 100644 index 0000000..ecc1fa7 Binary files /dev/null and b/img/blog/buzzfeed.jpg differ diff --git a/img/blog/cleverbot.jpg b/img/blog/cleverbot.jpg new file mode 100644 index 0000000..dda9504 Binary files /dev/null and b/img/blog/cleverbot.jpg differ diff --git a/img/blog/madlibs.jpg b/img/blog/madlibs.jpg new file mode 100644 index 0000000..5bdbde7 Binary files /dev/null and b/img/blog/madlibs.jpg differ diff --git a/img/blog/phone_keyboard.jpg b/img/blog/phone_keyboard.jpg new file mode 100644 index 0000000..293dc5c Binary files /dev/null and b/img/blog/phone_keyboard.jpg differ diff --git a/img/blog/spacy_speed.jpg b/img/blog/spacy_speed.jpg new file mode 100644 index 0000000..505d33c Binary files /dev/null and b/img/blog/spacy_speed.jpg differ